Contact Center AI:
29 Ways to Maximize Efficiency & Minimize Costs
Discover the Power of AI to Transform Your Contact Center’s Profitability
- Chapter I: Introduction & Definitions
- Chapter II: Enhancing Agent Productivity and Efficiency
- Chapter III: Improving Customer Experience and Engagement
- Chapter IV: Automated Quality Management, Agent Performance & Training
- Chapter V: AI-Powered Knowledge Management
- Chapter VI Data Analysis and Insights
- Chapter VII What’s Next For Contact Center AI?
- Chapter VIII FAQ: Implementing Generative AI In Contact Centers
Introduction & Definitions
AI has been used in contact centers for many years. In fact, MiaRec has been utilizing Machine Learning and Natural Language Processing for years to accurately transcribe call recordings, automatically extract keywords, and categorize calls by topics to humanize contact center data.
Despite the enormous impact Generative AI and other AI-based technologies will have on contact centers (for example, Gartner predicts that by 2025, “80% of customer service organizations will utilize generative AI to enhance agent productivity and customer experience”), many organizations struggle to envision exactly how they can benefit from this new technology.
In this resource, we will explain in non-techy terms what AI and related technologies are, and what you as a contact center manager have to know in order to confidently evaluate AI solutions for your organization. First, we outline 29 different use cases you should consider in your contact center.
What Are Generative AI & Conversational AI
Before we move on to how to use Artificial Intelligence in a contact center context, we have to define what Conversational and Generative AI are.
Generative AI
According to Gartner, Generative AI is the subset of Machine Learning that
“enables machines to learn from a representation of artifacts from data and models, and use it to generate brand-new, completely original artifacts that preserve a likeness to original data or models.
Generative AI can produce totally novel content (including text, images, video, audio, structures), computer code, synthetic data, workflows and models of physical objects. Generative AI also can be used in art, drug discovery or material design.”
In other words, Generative AI takes data inputs and generates new, original outputs based on those inputs, including writing text, composing music, creating artwork, evaluating new ideas, and much more.
In the Contact Center space, Generative AI technology is primarily used to generate summaries of meetings and telephone conversations, answer questions using knowledge base, extract information from texts, interpret text inputs and generate human-like responses.
Conversational AI
Conversational AI systems include chatbots and virtual agents that are able to interact with humans in a form of dialogue using natural language.
Contact centers use Conversational AI solutions to build virtual agents, chatbots or real-time agent assist systems. Modern Conversational AI systems use Large Language Models to achieve phenomenal results in the understanding of human language. The goal is to improve customer service experiences, make interfaces more user friendly, and human-like.
Differences Between Conversational AI and Generative AI
Both Conversational AI and Generative AI terms can be spotted in a description of the same product, but these terms are not interchangeable.
- Conversational AI usually describes an end product that provides chatbot or virtual agent capabilities.
- Generative AI term describes a technology that very often can be used inside the Conversational AI products to perform some of its functions. For example, chatbots can use ChatGPT (Generative AI technology) to generate human-like responses to human inputs.
If you hear “Conversational AI”, then most probably you can assume “Generative AI” is used in such a product under the hood, if the product is modern.
But, if you hear “Generative AI”, it doesn’t mean the product has “Conversational AI” capabilities. Generative AI technology can be used in other areas, for example, journalists can use it to refine their written texts, artists can use it to generate new art, etc.
Download This Guide
Download this comprehensive guide as a 40-page PDF document and read it later.
Enhancing Agent Productivity and Efficiency
Automating Post-Call Summary
When done manually, post-call wrap-up time can take, on average, anywhere from one to five minutes. Now multiply that by the 50 calls a contact center agent handles on a daily basis, and you are looking at 50 to 250 minutes of administrative time. Generative AI can streamline a lot of these post-call processes by automatically generating call summaries and disposition codes for CRM systems. This automation not only saves time but also standardizes summaries for future reference.
Examples: Five9 AI Summaries, MiaRec Auto Call Summary, Talkdesk Agent Assist, Salesforce Call Summaries
Image: Screenshot showing Five9 AI Summaries.
Image: Screenshot showing Talkdesk AI generated summary.
Image: Screenshot showing Salesforce Call Summaries powered by Einstein.
Automating Note Taking
Very similar to automatic post-call summary is Generative AI’s capability to automatically jot down important information DURING a customer call. This feature enables agents to better focus on the conversation as they no longer have to multitask between listening and note-taking. This tremendously improves call handling efficiency and helps prevent agent burn-out.
Example: Observe AI Auto Summary, Uniphore Agent Assist’s Summary & Disposition
Image: Screenshot of Observe AI Agent Assist
Example: Uniphore Agent Assist’s Summary & Disposition
Auto-Generating Customer Replies
Because Generative AI can understand intent, it can first read and understand a customer’s query and then try to automatically generate a helpful response by sifting through relevant sources like CRM systems, knowledge bases, customer databases, web links, and such. However, it is important that agents review and edit the responses before forwarding them to ensure their accuracy.
Examples: Salesforce, HubSpot, Talkdesk
Image: Example of Salesforce automated chat
Image: Example of the HubSpot chatbot builder
Image: Example of Talkdesk AI writes responses to customers
Auto-Completing Agents’ Responses
Solutions like Zendesk's "expanding agent replies" use Generative AI to complete responses for agents. Instead of just auto-completing his sentences like Google AutoComplete, these solutions will deploy AI to access various knowledge sources to finish an agent’s response, saving significant time in customer communication.
Example: Zendesk's "expanding agent replies"
Image: Screenshot of Zendesk expanding agent reply
Real-time Language Transcription and Translation
AI can provide real-time or near real-time speech-to-text transcriptions. You can also use Generative AI to instantly translate customer queries, enabling support in multiple languages and reducing language barriers. Real-time translations of customer conversation is huge as it allows an organization to broaden their customer base and enhance their global support capabilities. This is relatively common in text-based communication channels, but is starting to emerge in voice-based channels by augmenting your agents’ voices when translating.
Example: Parloa
Image: How Parloa real-time translation works
Streamlining Call Transfers and Escalations
Transferring and escalating calls is often time-consuming as it requires a knowledge transfer to update the agent overtaking the conversation. Generative AI tools can reduce wait times by summarizing conversations and transferring them to the next available support agent or supervisor. This reduces the need for customers to repeat their issues, streamlining the escalation process.
Example: Verint Interaction Transfer Bot
Image: Amazon Connect Catch up Summary during call transfer
Step-by-step Guidance of Agents in Real-time
An AI assistant can recommend the next best action in real-time based on conversation.
Example: Uniphore’s U-Assist, Five9 Agent Assist with Real-Time Guidance
Image: Uniphore’s U-Assist agent assisted real time coaching
Image: Five9's Agent Assist giving real-time guidance
Searching Knowledge Base for an Answer to Customer’s Questions
AI-based assistants can automatically find answers to customer’s questions, eliminating frustrating and tedious manual searches. This is leading to an increase in first-call resolution and a decrease in agent errors. It can also improve customer experiences as the agent becomes more in tune with the customer’s concerns and has solutions at hand to resolve the problem right away, without putting the customer on hold or having to transfer them.
Example: Uniphore Agent Assist, Genesys Agent Assist
Image: Uniphore's Agent Assist with real-time coaching
Image: Genesys chatbot using knowledge base answers
Post-Call Agent Coaching
You can also use Generative AI to offer automated performance summaries after a call has ended. These summaries can be used to provide feedback and develop improvement strategies for agents, thus enhancing their performance and the overall customer experience.
Example: Manager Assist for Amazon Connect, MiaRec Assist
Image: Amazon Connect’s Manager Assist
Image: MiaRec’s Agent Assist auto call summary example
Automating processes
Where an AI Assistant accurately predicts the next best action(s) for the interaction, the process automation robot can trigger backend tasks on behalf of the agent, saving valuable time and eliminating manual errors.
Example: Uniphore robotic process automation
Image: Uniphore's robotic triggering of tasks in the backend
Download This Guide
Download this comprehensive guide as a 40-page PDF document and read it later.
Improving Customer Experience and Engagement
Generative AI plays a crucial role in improving customer experience and engagement in contact centers by providing personalized, efficient, and empathetic interactions.
Empower Self-Service through AI-powered Chatbots and Virtual Assistants
One of the maybe most straight-forward applications of Generative AI is the implementation of chatbots and virtual assistants for self-service. They enable customers to independently address common queries by typing in their question or concern into a chatbot or conversational interface. This allows support teams to focus on more complex issues, reducing hold times, lowering Average Handling Time (AHT), and increasing customer satisfaction.
Example: Amazon Lex Chatbot
Image: Lex chatbot on mobile
Example: HubSpot Chatbot, TalkDesk, Vonage
Image: TalkDesk chatbot with dashboard
Improving Self-Service Calling Experience
AI-powered Conversational Interactive Voice Response (IVR) uses Natural Language Processing (NLP) to ingest, analyze, and understand customer requests and then provide an automated response without the help of a live agent. It can create personalized experiences and, if needed, will direct customers to the appropriate self-service or agent channels based on their stated needs.
Example: GetVOIP, Teneo.ai, Five9 Intelligent Virtual Agent
Image: GetVOIP IVR call flow
Understanding Customer Sentiment
Another common use case for Conversational and Generative AI is analyzing customers' sentiments, moods, and emotions during interactions. This can be used live to gauge appropriate reactions, or be used post-call for quality management. For example, measuring how well an agent can navigate difficult conversations (angry customer = negative sentiment score) and bring them to a good outcome (happy customer = positive sentiment score) can give you real-world insights into an agent’s people skills and emotional intelligence. This helps to foresee and prevent escalations, improve satisfaction, and reduce churn rates.
Example: MiaRec Sentiment Analysis
Image: MiaRec's dashboard on sentiment analysis
Identify Potential Growth Opportunities
By analyzing conversation data, Generative AI can identify potential issues or growth/upsell opportunities (e.g., increased calls about shipping problems, calls requesting a feature that is not available) proactively. Essentially, AI mines your customer interactions to determine the interactive drivers, and surfaces these insights as trends or shifts in distribution.
Example: MiaRec Topic Analytics, Five9 Insights using OpenAI
Image: Miarec’s topic analytics dashboard
Download This Guide
Download this comprehensive guide as a 40-page PDF document and read it later.
Automated Quality Management, Agent Performance & Training
Automated Agent Evaluation and Call Scoring
Rather than manually evaluating a small fraction of calls at random, Generative AI can be used to automatically score 100% of your calls, providing a much more accurate representation of agent performance over time. These so-called Auto QA tools free up a tremendous amount of time every week that can be used in higher-value activities, such as focusing on calls flagged for follow-up or review.
Example: MiaRec Auto Call Scoring
MiaRec’s Auto Score Card
Adding Context to Automated Quality Scoring
For some questions like “Did the agent say their name?” the answer is simply a yes or a no, while answering questions like “Was the agent polite?” requires more context. While Generative AI can be used to automatically evaluate the call and gauge the level of politeness, it can also provide context as to why the answer might be “no” by including a quick statement about how the agent responded rudely to the customer’s request for a refund. This gives the supervisor enough context to dig deeper if needed.
Example: MiaRec Auto Call Scoring
Image: MiaRec's auto call scoring adding context
Identifying Agent Training Opportunities
Similarly to post-call coaching, Generative AI can identify opportunities for improvement and make individualized and unbiased suggestions for additional training based on the call evaluation to enhance the agent’s performance.
Example: MiaRec Auto Call Scoring
Image: MiaRec's auto call scoring identifying training opportunities
Alerting Supervisors to Issues With Their Agents
As a contact center supervisor, you always want to know if something extraordinary happens with your agents. Maybe your agent is struggling with a personal crisis and comes to work sleep-deprived and in a foul mood, which he takes out on the customers he or she deals with. Generative AI can identify if swear words are being used, if the mood in the call suddenly goes icy (sentiment analysis), or if the customer gets angry. As a supervisor, you will be notified and can intervene as soon as possible.
Example: NICE Enlighten Actions, MiaRec Auto QA + Notifications
Image: NICE Enlighten Actions dashboard
Image: Example of an automated agent scorecard from MiaRec
Automating Compliance Management (Data Redaction)
Contact centers process an enormous amount of Personal Identifiable Information (PII) and payment card numbers on a daily basis. Those get stored in your call recordings and the associated transcripts. In most cases, you are obligated by regulatory compliance to redact them. Machine Learning and Named Entity Recognition (NER) can reliably identify and redact sensitive information from your calls and transcripts, increasing your compliance and decreasing your liability should you suffer a data breach.
Example: MiaRec Auto Redaction
Image: MiaRec’s auto redaction in a call transcript
Supporting content
- MiaRec Auto Score Card
- Auto Redaction
- 7 Misconceptions about AI-Based Auto QM in Contact Centers
- New Automated QA Feature Update Helps You Improve Auto Score Card Accuracy
- Introducing MiaRec's Auto Score Card: Evaluate Agent Performance On 100% Of Your Calls
- Auto QA ROI Calculator
- MiaRec Takes Auto Data Redaction To New Heights With Machine Learning
AI-Powered Knowledge Management
The long-term goal of utilizing Generative AI in your contact center is to automate as many calls and inquiries as possible by providing the answer in an external- or internal-facing knowledge base and having an AI-based chatbot or virtual assistant answer them. The following five use cases help you get there.
Spotting Gaps In the Knowledge Base
Generative AI can identify when there are gaps in a contact center's knowledge base, flagging them to management for the creation of new knowledge articles or improving existing articles based on the collected feedback.
Example: TalkDesk Knowledge Management
Image: TalkDesk Knowledge Management spotting gaps
Other Examples: Google CCAI Generative FAQ, Twilio CustomerAI, Verint Knowledge Creation and Suggestion Bots
Generating Knowledge Base Articles
After it has identified gaps, Generative AI can also be used to fill these gaps by creating new knowledge articles. It can do this by understanding the customer intent and analyzing how agents and supervisors have successfully handled similar queries in the past. But as always, be the human in the loop and review and edit anything before it gets published.
Example: Twilio CustomerAI, Verint Knowledge Creation Bot
Image: AI generated knowledge base
Generating FAQs from Customer Conversations
This involves AI tools analyzing customer conversations to generate FAQs, helping contact centers better understand and address common customer queries.
Example: Google CCAI Generative FAQ
Chat-Like Information Discovery
Rather than having to read help articles in the knowledge base with the customer waiting on hold, the agent can use AI-enhanced search. Essentially, an agent writes a quick query and Generative AI augmented search capabilities will auto-generate the most probable answer based on the internal knowledge base. This not only improves access to information in knowledge bases, but also makes knowledge discovery much faster and easier.
Example: Uniphore Agent Assist with Knowledge Base search
Image: Uniphore's Agent Assist recommendations
Data Analysis and Insights
Generative AI can provide you with powerful insights and help you discover trends in your consumer behavior, preferences, and intent.
Call and Trend Analytics
AI can provide more comprehensive call analytics and identify trends more accurately than a human customer support manager because it tracks customer sentiment, tone, and personality, and it has a much more complete picture of what is going on across all calls. It is often utilized for in-depth analytics on various aspects of calls, such as call times and first resolution rates.
Example: MiaRec Sentiment Trending Dashboard
Image: Screenshot of MiaRec's Sentiment Trend dashboard
Automatic Keyword Spotting
One of the easiest but nevertheless powerful applications of generative AI is to automatically spot certain keywords in call transcripts to identify calls that mention competitors, brand or product names, specific features, marketing campaigns or similar information. This can offer valuable insights into customer preferences and market trends.
Example: MiaRec Voice Analytics
Image: A sample sentiment score from MiaRec's Voice Analytics
Discover Call Reasons and Extract Customer FAQs
The ability of Generative AI to uncover customer intent and categorize primary reasons why your customers contact your business is very helpful in getting better insight into call reasons. This helps in understanding demand drivers and prioritizing actions to improve contact center operations. In addition, you can extract frequently asked questions and answer them in your knowledge base.
Example: MiaRec Voice Analytics, Google Cloud’s Generative FAQ for CCAI Insights
Image: Call reason trend report in MiaRec
Extracting Insights from Customer Feedback
Generative AI solutions can ingest and analyze large amounts of customer feedback from various sources (such as call transcripts, surveys, and online reviews), distill them into structured, actionable insights, and enable continuous monitoring and quick responses to emerging issues.
Example: Verint Insights Bot
Identifying What Makes Conversations Successful & Define Resolution Paths
Generative AI can assess thousands of customer call transcripts and assess which ones are the most successful conversations. Then it can identify what worked and what didn’t, resulting in ideal paths for call resolution. This is invaluable insight that informs agent coaching and scorecard creation initiatives.
Example: Cresta Flow Modelling
Measuring The Performance of Marketing Campaigns
Generative AI tools can track and analyze customer interactions to assess the effectiveness of marketing campaigns, providing insights into customer engagement and responses to marketing efforts. In the simplest case, the marketing campaign has a specific coupon code the customer needs to mention when placing an order or booking a service that is then recognized as a keyword to be tracked. Or you can measure an uptake in interest in a specific product or service that has been recently promoted.
Example: MiaRec Voice Analytics
Image: Measuring marketing campaigns with MiaRec's call summary
Supporting content
- Call Trend Analytics
- Automatic Keyword Spotting
- AI-Powered Call Types Analysis
Download This Guide
Download this comprehensive guide as a 40-page PDF document and read it later.
What’s Next For Contact Center AI?
Compared to any other technical evolution or innovation in contact center technology, Contact Center AI is developing at an unprecedented velocity. It is hard to predict what it will look like in three to five years from now due to the sheer speed of development.
Here at MiaRec, we have deployed Machine Learning, Large Language Models, and Generative AI for years, and the analysis below is maybe more grounded and less over-hyped than you might see in the media. We strongly feel that it is important to educate the market on what is realistic and possible. Having said that, here is what we do know regarding where Contact Center AI will be heading in the next 12-24 months.
Generative AI Will Have To Pass Through The “Trough Of Disillusionment” First
In its Hype Cycle for Customer Service and Support Technologies 2023 report, the analyst company Gartner places Generative AI right at the peak of “Inflated Expectations” and on the brink of its descent into the infamous “Trough of Disillusionment.” If you are unfamiliar with the Gartner Hype Cycle concept, don’t worry. Every new technology goes through this hype cycle as it matures.
In this case, this means that there are a LOT of unrealistic expectations fueled by even more marketing hype. A lot of vendors we have investigated for this document are in the beginning stages of developing the product they are promoting on their website, and some solutions even only exist as a press release and an Adobe Illustrator-created screenshot mock-up.
Contact Center AI Will Become More Powerful & Affordable As It Matures
As it passes through the “Trough of Disillusionment,” the market will adjust its expectations to more realistic goals, and Generative AI-based Contact Center solutions will become more powerful and feature-rich. For example:
- Contact Center AI will continuously improve in accuracy, especially in real-time analytics, and speed (e.g., real-time transcription),
- AI-powered assistants will become more powerful, helping contact center agents to handle calls and chats more efficiently, and improving managers’ performances,
- Generative AI will answer customer questions on the spot using a knowledge base which will help to reduce training time for agents and call-handling time, as well as improve customer satisfaction, and
- AI-driven chatbots will increasingly become smarter and more capable of doing more complex tasks.
In addition to the functionality enhancements, the costs of AI-based solutions will decrease overtime, making it affordable to all customers.
Contact Centers Will Look To Generative AI To Improve Agent Productivity & Customer Experience (CX)
Despite all the disillusionment, Contact Center AI holds tremendous benefits, cost saving potential, and productivity gains for those who adopt it. In the next 24 months, we expect a huge shift in Contact Center AI adoption and we aren’t the only ones. Gartner predicts that by 2025, “80% of customer service and support organizations will be applying generative AI technology in some form to improve agent productivity and customer experience (CX).” In other words, if you aren’t already evaluating Contact Center AI, you need to start ASAP!
Deeper Integration & Automation Of Back-End Tasks
One often ignored aspect of further development in terms of Contact Center AI is how it will impact the back-end. As the technology matures, integration of AI tools with external systems will become tighter. One example is Generative AI recognizing a knowledge gap and injecting data into the knowledge base from various internal sources. Another example is the ability to automate back-end tasks, i.e., canceling shipments with one-click, resulting in a massive reduction of manual work that agents have to perform.
Easy & Fast Data Mining To Uncover Customer Insights Becomes Commonplace
A currently unexplored area is Generative AI’s capability to facilitate easy-to-use and fast data mining across all recordings. End users will be able to use a ChatGPT-like interface to ask simple questions like “What are the most common reasons for canceling appointments?” or “What are the top 3 products customers called about in the last 12 months?”
Currently, to answer such questions, the system must be manually pre-configured to extract specific data insights. It may involve a custom model training, which is expensive and time consuming. In the future, users will be able to ask free-form questions, and the AI-based system will find answers to them in millions of recordings easily.
In addition, decision makers will be able to use data buried in call recordings to inform business decisions. At the moment, the recordings are used in contact centers primarily for evaluating agent performance. In the future, data from call recordings could be made available to other departments for mining and extracting insights. For example, a purchasing manager of an online retail company may ask an AI assistant to name a product that callers are interested in buying, but the company doesn’t offer yet. Now, agents simply say “We don’t sell XYZ products,” and such a lost opportunity is not tracked anywhere.
Book A Demo Today
Book a personalized product demonstration to learn how you can transform your contact center with AI.
FAQ: Implementing Generative AI In Contact Centers
1. What are the key benefits of implementing Generative AI-based solutions in our contact center?
Generative AI can enhance customer experience, improve agent efficiency, reduce response times, and provide valuable insights from customer interactions.
2. How will Generative AI integration impact our current workforce?
Generative AI is designed to augment the capabilities of your human workforce, not replace them. Think of Generative AI as something that makes you more efficient and takes care of all the tedious manual tasks. It can also assist agents with real-time recommendations and automate routine tasks, allowing them to focus on more complex customer needs.
3. What is the typical cost and ROI of implementing Generative AI solutions?
Costs can vary based on the scale and complexity of the implementation. However, the ROI often manifests itself in improved customer satisfaction, higher agent productivity, and reduced operational costs. We have created two ROI calculators for specific AI features that will give you a good indication of what to expect: the Auto Call Summary ROI Calculator and the Auto QA ROI Calculator.
4. What are the data privacy and security implications of using Generative AI?
Implementing Generative AI requires adherence to data privacy laws and regulations. It's crucial to ensure that the AI solutions are compliant with GDPR, CCPA, and other relevant data protection frameworks.
5. How can we ensure our Generative AI system remains up-to-date and effective?
It is important to understand that AI gets better over time as it learns and improves itself based on the data inputs it receives. Regular training and updates are therefore essential. This involves feeding the AI system with new data, adjusting algorithms based on performance analytics, and staying updated with the latest AI advancements.
6. Can Generative AI be integrated with our existing CRM and IT infrastructure?
Yes, most Generative AI solutions are designed to be compatible with existing CRM systems and IT infrastructure. However, a detailed assessment by your IT team is advisable to ensure seamless integration.
7. How does Generative AI handle complex or sensitive customer inquiries?
For complex or sensitive issues, Generative AI can flag these inquiries to be handled by human agents. It's also capable of learning from such interactions to handle similar future queries more effectively.
8. What training is required for our team to effectively use Generative AI tools?
Training varies depending on the complexity of the tool. It generally includes understanding the AI interface, learning how to interpret AI recommendations, and training on data input and system monitoring. For now, most AI use cases can be achieved without having to hire a data scientist. However, as your AI requirements and solutions evolve, you will need qualified personnel.
9. How do we measure the success of a Generative AI implementation in our contact center?
Success can be measured through various KPIs like customer satisfaction scores, average handling time, first call resolution rate, and overall cost savings.
10. What kind of customer experience improvements can we expect with Generative AI?
You can expect more personalized interactions, quicker resolution times, and enhanced self-service options, leading to an overall improved customer experience.
11. Are there any industry-specific considerations we should be aware of when implementing Generative AI?
Yes, different industries might have specific compliance, language, and customer interaction nuances. It's important to choose a Generative AI solution that can be tailored to meet these specific industry requirements.
12. How long does it typically take to implement Generative AI in a contact center?
The implementation timeline can vary, ranging from a few hours to several weeks or even months, depending on the scale of the project and the complexity of your existing systems.
From Our Blog
Stay up to date with what is new in our industry, learn more about the upcoming products and events.
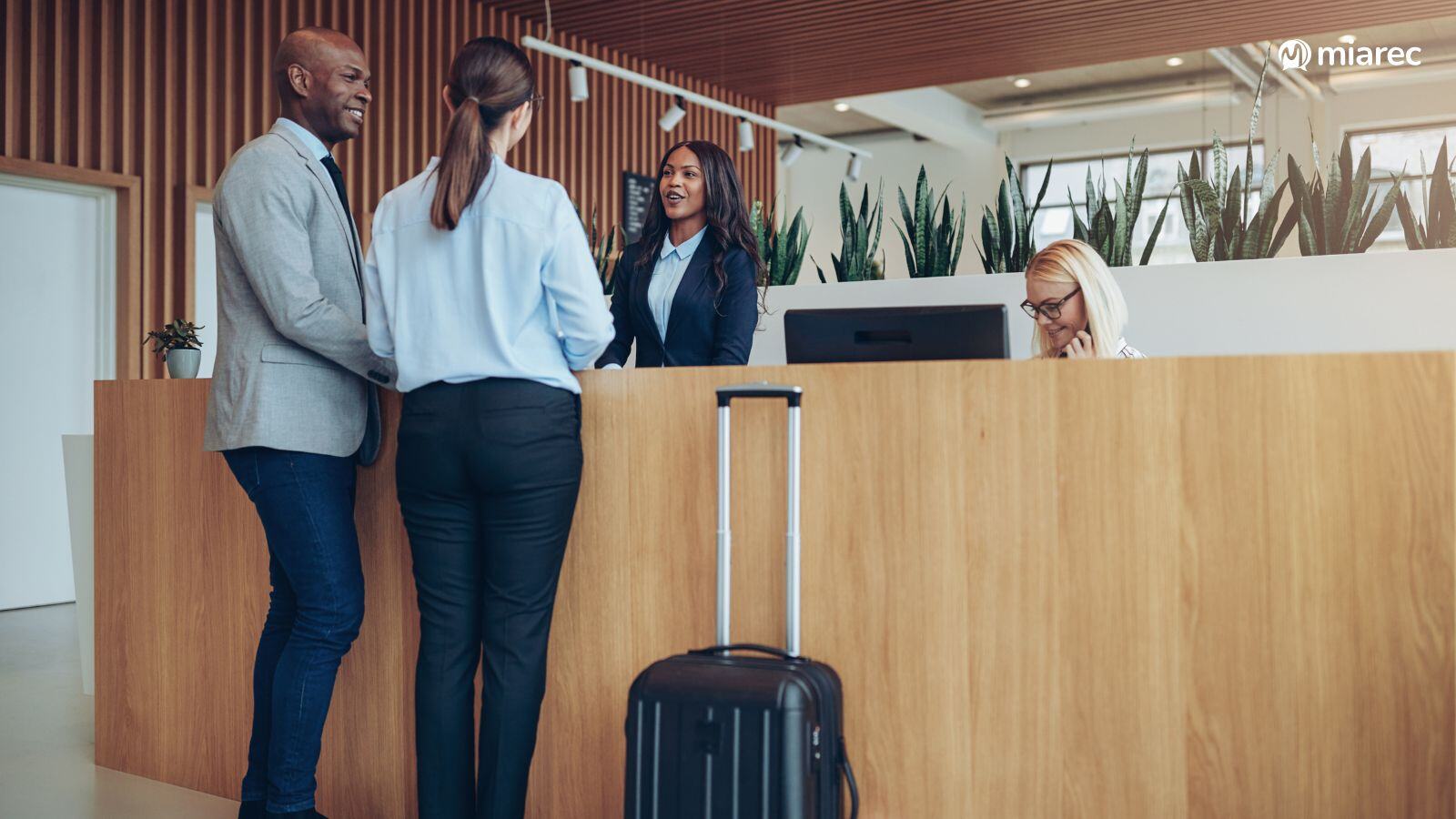
6 Ways To Boost Your Hospitality Contact Center with Generative AI
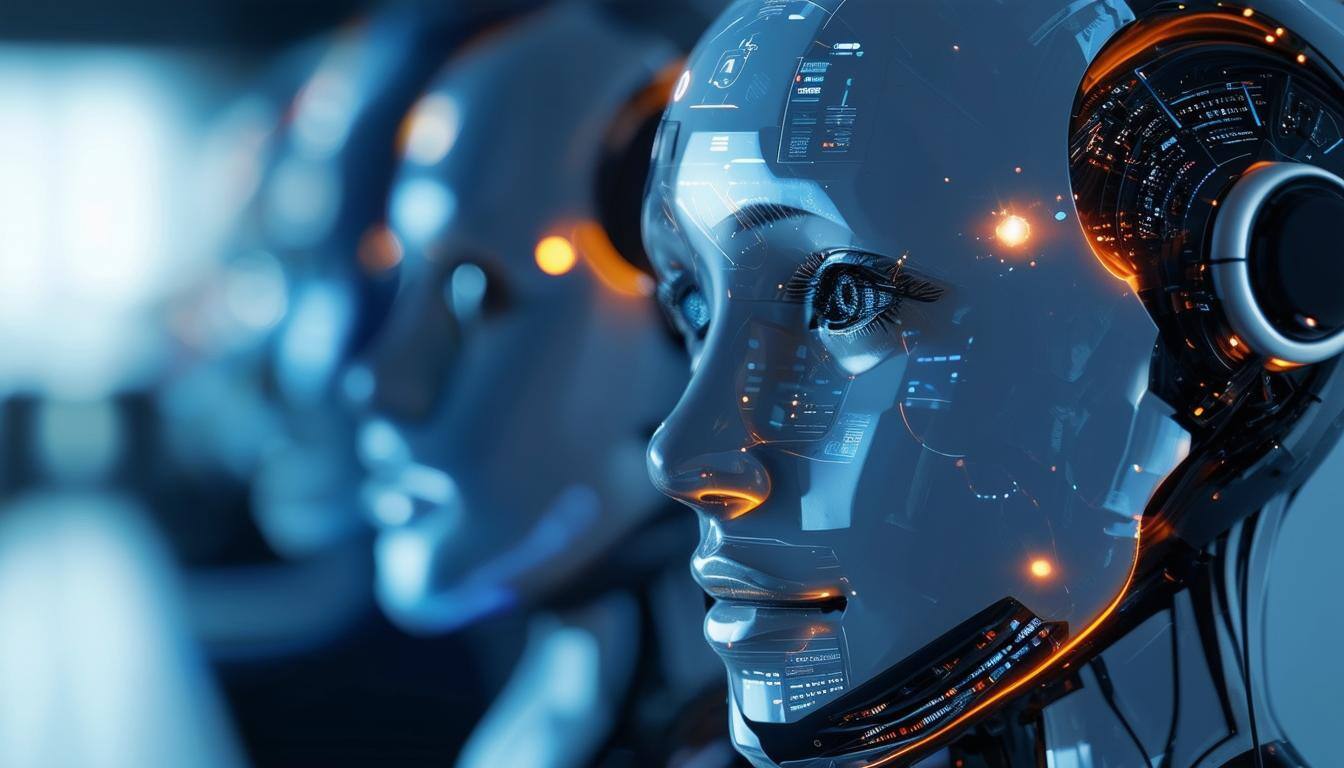
Press 0 for the Future: Voice AI Agents Set to Transform Contact Centers in 2025
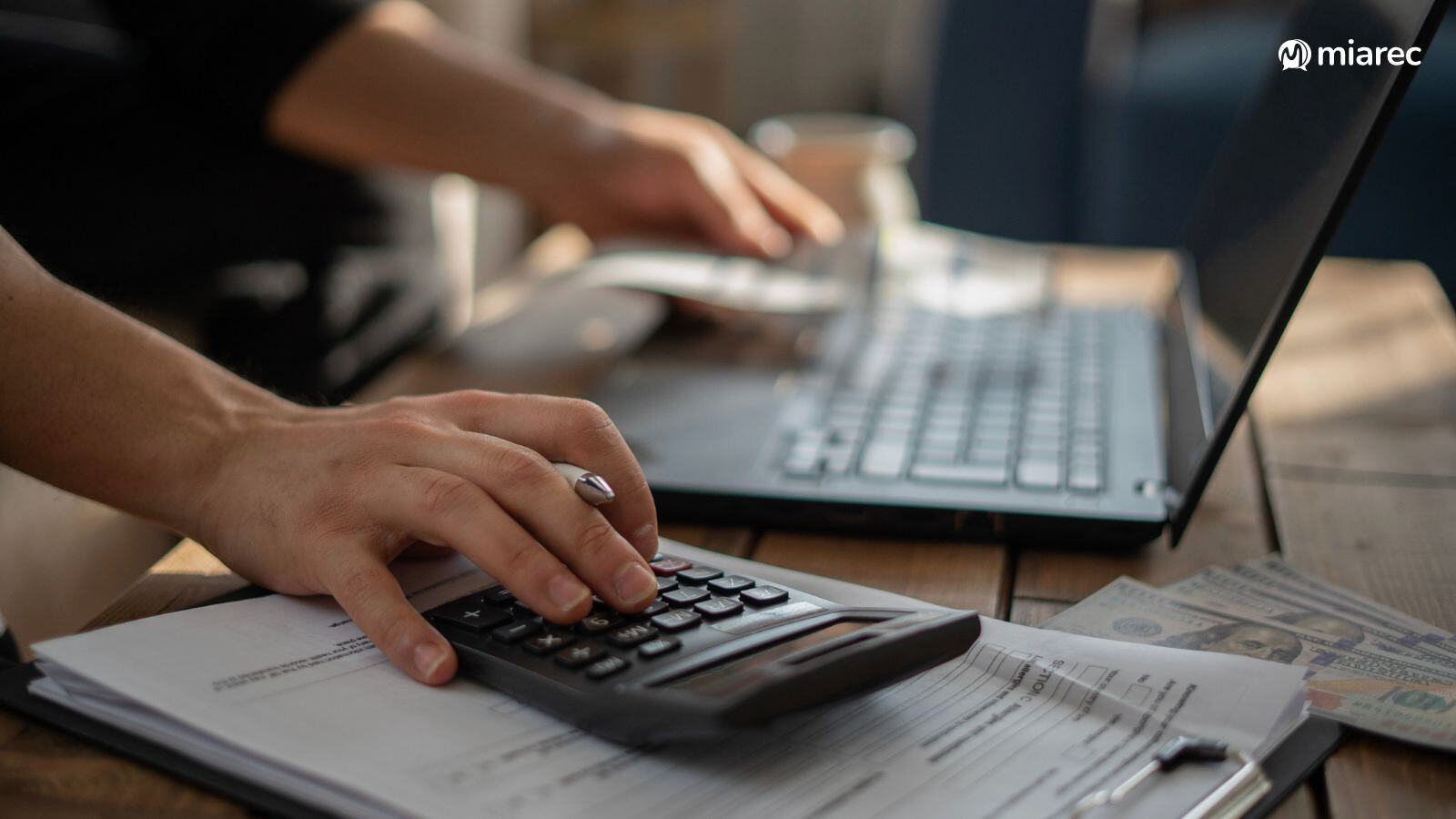